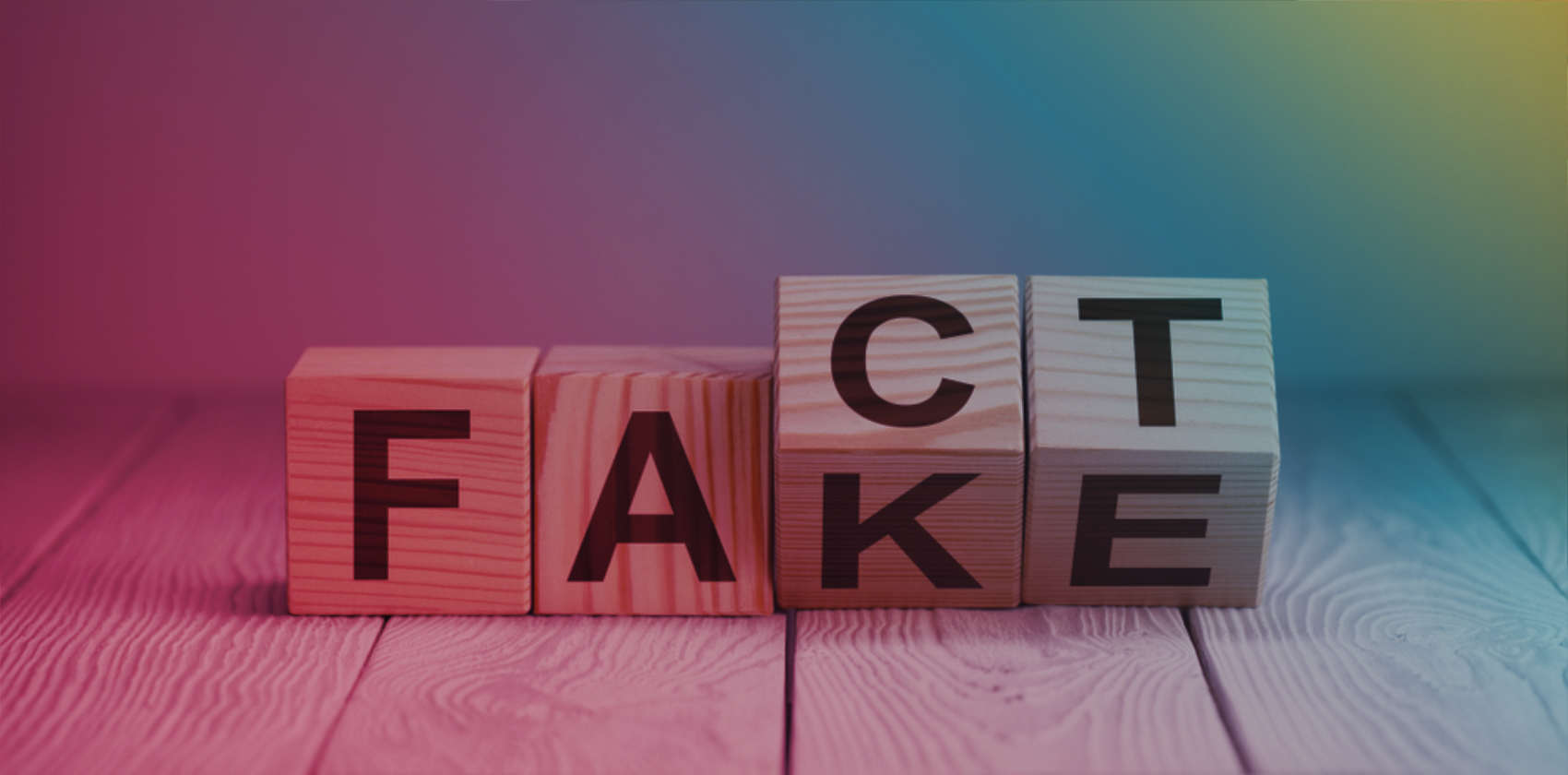
Liability for AI-generated Content
This article explores liability for text and image content produced by generative AI. In the case of wrong output, or hate speech/toxic output – who should be responsible (given the many parties involved in developing and deploying the AI system and the user also influences the output by inputting the prompt), how are the industry and the regulators reacting, and is it enough? An earlier article discussed the IP issues arising from the content produced, and this article now examines the nature of the content. This article is timely in light of the spate of regulatory and industry developments this year – Singapore has released a model governance framework for generative AI for public consultation in January, an airline has recently argued that its chatbot should be responsible for its own actions, and the industry has enhanced its offerings with hyper-realistic text-to-video (Open AI’s SORA), amplifying the challenges of deepfakes and disinformation.
Generative AI output can range from the hilarious to the hateful to the hurtful. But who should be responsible for the output of generative AI where the content is1The six risks posed by generative AI were identified and discussed in the Infocomm Media Development Authority’s paper on “Generative AI: Implications for Trust and Governance” published on 6 June 2023 (“Generative AI Risks Paper”), affirmed again by ASEAN in February 2024 in the ASEAN Guide on AI Governance and Ethics – of which this article will focus on these 2 risks. –
- [Part 1] Factually incorrect – i.e. mistakes and “hallucinations”2This is the first risk identified in IMDA’s Generative AI Risks Paper, which acknowledges that all generative AI models will make mistakes (page 9). (where the output ranges from “plausible-sounding but factually incorrect”,3As described in Google’s model limitations for its generative AI products, available at https://cloud.google.com/vertex-ai/generative-ai/docs/learn/responsible-ai to completely nonsensical); or
- [Part 2] Toxic – e.g. profanities, identity attacks, sexually explicit content, demeaning language, and language that increases violence?4This is the third risk identified in IMDA’s Generative AI Risks Paper, which remains a challenge so long as generative models “mirror language from the web” (page 11).
Given the way generative AI models work, a human is not entirely in control of the output, regardless of whether they are the developer who trained and coded the AI model, or the user who entered the prompt. Large Language Models (LLMs) generate text based on the probability of what word comes next in a sequence of words,5For information about how LLMs work, read more at https://developers.google.com/machine-learning/resources/intro-llms. and text-to-image generators also operate with an element of randomness as they “[do] not treat text prompts as direct instructions, [so] users may need to attempt hundreds of iterations before landing upon an image they find satisfactory”.6See the US Copyright Review Board’s decision on Théâtre D’opéra Spatial dated 5 September 2023, in particular pages 6 and 7, available at https://www.copyright.gov/rulings-filings/review-board/docs/Theatre-Dopera-Spatial.pdf. This is in contrast to someone who personally writes a chunk of text or designs an image – the words/picture that comes out is exactly what they intended.
Therefore, who should be liable for the wrong or toxic output produced by generative AI (if anyone is to be responsible at all):
- the developer – the entity that designs, codes or produces the AI system7The definition of “developer” is taken from the ASEAN Guide on AI Governance and Ethics (page 9). (e.g. OpenAI who developed the LLM that powers ChatGPT). The developer may not have control over how its AI system is subsequently deployed by another company;8See the distinction between AI developers and deployers at https://www.bsa.org/files/policy-filings/03162023aidevdep.pdf.
- the deployer – the entity that uses or implements an AI system in a particular scenario, which could be developed by their in-house team (in which case the entity is also the developer), or via a third-party developer9The definition of “deployer” is adapted from the ASEAN Guide on AI Governance and Ethics (page 9). (e.g. a company puts an AI-enabled chatbot on its website for visitors to interact with);10Please note that an in-depth look into how to allocate liability as between developer and deployer is outside the scope of this article (in order to remain within the word limit). However, the following is a useful resource on the allocation of liability depending on how the model is made accessible to a developer downstream (via API or open source): https://www.adalovelaceinstitute.org/blog/value-chain-general-purpose-ai/. Singapore’s proposed Model AI Governance Framework for Generative AI (issued on 16 January 2024) also puts forth the proposition that “responsibility can be allocated based on the level of control that each stakeholder has in the generative AI development chain, so that the able party takes necessary action to protect end-users.” (p. 6).
- the user (or prompter) – the person who enters the prompt into the generative AI system and gets the output/result.11The definition of “user” is adapted from the ASEAN Guide on AI Governance and Ethics (page 9), but in this case there is no “decision” made by the generative AI system. The answer is not always straightforward because the output is in part determined by what was the prompt – if a user deliberately crafts a prompt to manipulate the system to breach its guardrails and produce erroneous or toxic content, to what extent is the developer/deployer absolved from liability?12This excludes scenarios where the user is legitimately engaged by the developer or deployer to test the AI system (e.g. red-teaming).
The act for which liability will attach will differ as between developer, deployer and user:
- for the user, it would be either or both:
- taking the text/image generated and making it public (i.e. letting persons other than himself/herself see it);
- deliberately prompting for the toxic or false/erroneous text/image – thereby having a part to play in the outcome generated and possibly reducing or eliminating liability for the developer/deployer;
- for the developer/deployer, it would be for developing or releasing a product that has a known propensity to give false/erroneous or toxic output.
In the case of the user, if he/she subsequently takes the AI-generated output and makes it public (e.g. publishes it online), he/she may potentially be liable if the content was false, defamatory or was illegal/hate speech,13Potential laws that could apply would include the Penal Code 1871 (e.g. for distributing intimate images, or for cheating (e.g. a deepfake is used in scams)); the Protection from Harassment Act 2014, the Undesirable Publications Act 1967 and the Protection from Online Falsehoods and Manipulation Act 2019 (“POFMA”). as if he/she had come up with the image or text himself/herself. This is because by posting the content he/she would be taken to have affirmed that speech or image. A user may have limited success in pleading that they did not know the content was false if they were warned by the LLM developer/deployer that the LLM does not always produce accurate content.14Separately, see also section 11(4) of the POFMA where a person who communicates a false statement of fact in Singapore may be issued a Correction Direction even if the person does not know or has no reason to believe that the statement is false. The High Court in The Online Citizen Pte Ltd v Attorney-General (2020) SGHC 36 explained that “Section 11(4) of the POFMA is intended to prevent and stop the spread of falsehoods and misleading information when information is posted online without prior verification and thought, be it deliberate or otherwise.”
But taking a step back, we have to look at how the wrong or toxic output came about in the first place, so the bulk of this article will focus on the liability of the developer/deployer.
Both factually inaccurate and toxic output cause different levels of harm and the solutions to tackle them also differ. Therefore, we will discuss the existing solutions from regulators and the industry, and evaluate their sufficiency. A key question to address is whether adopting these solutions — e.g. (1) deploying the latest technological solutions (like Retrieval-Augmented Generation and content filters) and (2) warning users that output may still be inaccurate/toxic — is sufficient to negate any liability that may arise.
Part 1: Liability for Incorrect Output (Hallucinations) – This Part is Exclusive to LLMs
LLMs have given made-up answers, which can catch the unwary who do not check its output (recall the lawyer who submitted made up cases to the court when relying on ChatGPT to write his brief)15See Roberto Mata v Avianca, Inc., as reported in https://www.theverge.com/2023/5/27/23739913/chatgpt-ai-lawsuit-avianca-airlines-chatbot-research, or have a harmful effect on a person’s reputation:
- March 2023: an Australian mayor threatened to sue OpenAI for defamation because when asked “what role did Brian Hood have in the Securency bribery saga”,16As reported in https://www.smh.com.au/technology/australian-whistleblower-to-test-whether-chatgpt-can-be-sued-for-lying-20230405-p5cy9b.html ChatGPT replied that he was convicted in the bribery case (which was untrue – he was the whistleblower);
- April 2023: in response to a prompt to cite examples of sexual harassment by professors at American law schools, ChatGPT gave false output that professor Jonathan Turley was accused of sexual misconduct and even supplied a fake article from the Washington Post in support of the allegations;17As reported in https://nypost.com/2023/04/07/chatgpt-falsely-accuses-law-professor-of-sexual-assault/
- June 2023: Mark Walters filed a lawsuit against OpenAI for defamation when a journalist asked ChatGPT to summarise a case involving the Second Amendment Foundation, and ChatGPT responded that Mr Walters had embezzled funds from the Foundation and even came up with fictitious passages from the case;18https://nypost.com/2023/06/07/mark-walters-suing-chatgpt-for-embezzled-hallucination/, where the New York Post also described it as the “first-ever defamation lawsuit” against OpenAI.
In the hands of a user/prompter who knows that he/she must check the output and knows how to check the output, the impact of wrong answers is not so serious. The British judge who used ChatGPT to write a judgment gave it a task “which [he] knew the answer and could recognise as being acceptable”,19As quoted in https://www.straitstimes.com/world/europe/british-judge-taps-jolly-useful-chatgpt-for-part-of-his-ruling#:~:text=A%20British%20Court%20of%20Appeal,Lord%20Justice%20Colin%20Birss%20said. and described ChatGPT as “jolly useful”.20As quoted in https://www.straitstimes.com/world/europe/british-judge-taps-jolly-useful-chatgpt-for-part-of-his-ruling#:~:text=A%20British%20Court%20of%20Appeal,Lord%20Justice%20Colin%20Birss%20said.
However, if the generated answer goes to the user/prompter directly in a situation where the user/prompter is not reasonably expected to verify the correctness of the answer on their own – the wrong answer can have serious consequences. This is where solutions are urgently needed to reduce such instances of wrong answers.
What are the Current Solutions?
#1: Retrieval augmented generation and other technological solutions21There are many more technological solutions, but I will focus on those mentioned in discussion papers issued by IMDA to date, namely the Generative AI Risks Paper (issued in June 2023), the Cataloguing LLM Evaluations paper (issued in October 2023), and the proposed Model AI Governance Framework for Generative AI (issued on 16 January 2024).
On the technology front, Singapore’s proposed Model AI Governance Framework for Generative AI (Model Gen-AI Framework) recommends techniques such as Retrieval-Augmented Generation (RAG) and few-shot learning to reduce hallucinations.22See page 19 of the Model Gen-AI Framework: “For example, further fine-tuning or using user interaction techniques (such as input and output filters) can help to reduce harmful output. Techniques like Retrieval-Augmented Generation (RAG) and few-shot learning are also commonly used to reduce hallucinations and improve accuracy.”
With RAG, instead of merely entering a prompt which goes to the LLM, the prompt is paired with information retrieved from a repository/database of information (which the user can curate) that is relevant to the content of the prompt, before it goes to the LLM. The LLM can then draw on the material to anchor its response. To illustrate RAG in layman terms, if you merely prompt ChatGPT to give you a new recipe to try, you might get something nonsensical like “banana spinach jelly”, but if you ask it for a new recipe and a few of your favourite recipes are pulled from the repository and sent together with the prompt to the LLM, you are more likely to get back something tasty. 23Adapting the example provided by Moveworks in https://www.youtube.com/watch?v=6dxkBftbukI.
Second, using Reinforcement Learning through Human Feedback, where humans give feedback on the model’s responses and the model then incorporates the feedback to improve the content it generates, has also proven to be effective in reducing hallucinations and toxic content generated.24See page 13 of IMDA’s Generative AI Risks Paper, which states that with RHLF, “language models learn to follow instructions better and generate results that show fewer instances of “hallucination” and toxicity (even though bias still remains as an open problem)”. See also the concept of “chain-of-thought prompting” mentioned in page 22 of IMDA’s Generative AI Risks Paper, as a means to improve model quality with better explainability through reasoning. It involves providing the LLM with a question and also the method to solve it, so that the LLM will not only give the answer but also show its reasoning for the answer, increasing the likelihood of getting the correct answer – read more about chain-of-thought prompting at https://blog.research.google/2022/05/language-models-perform-reasoning-via.html.
However, it is widely acknowledged that these methods cannot eliminate the risk of hallucinations entirely.25See, for example, the Generative AI framework for HM Government created by the UK’s Central Digital and Data Office at page 10, accessible at: https://assets.publishing.service.gov.uk/media/65c3b5d628a4a00012d2ba5c/6.8558_CO_Generative_AI_Framework_Report_v7_WEB.pdf. See also the interviews at https://fortune.com/2023/08/01/can-ai-chatgpt-hallucinations-be-fixed-experts-doubt-altman-openai/.
#2: Warning users that LLMs hallucinate so that they will check the output
The industry practice is that providers of generative AI services also have disclaimers, warning that the service may provide “inaccurate or offensive” 26See, for example, Google’s Disclaimer at https://cloud.google.com/terms/service-terms, which states that “Generative AI Services use emerging technology, may provide inaccurate or offensive Generated Output, and are not designed for or intended to meet Customer’s regulatory, legal or other obligations.” output. This was in fact what OpenAI relied on in its motion to dismiss the Mark Walters defamation case – it had already warned users that its product could produce inaccurate information.27As reported in https://futurism.com/the-byte/judge-refuses-dismiss-libel-suit-openai and https://www.insideradio.com/free/another-setback-for-openai-in-radio-hosts-defamation-suit/article_ffb78558-b583-11ee-a92d-53d6ae459450.html It remains to be seen how effective this defence will be as the case develops.
However, some take the view that LLM developers can’t have it both ways because they promote the reliability of their models (e.g. passing bar exams), and at the same time they say that they hallucinate.28Eugene Volokh, Professor of Law at UCLA School of Law, in “Large Libel Models? Liability for AI Output” published in the Journal of Free Speech Law, August 2023 (at pages 501 and 498 to 499). Furthermore, a disclaimer would only be effective against the user (who can’t then commence action for receiving and relying on the wrong output), but it does not waive the right of a third party who was the subject of a defamatory statement to sue.29Eugene Volokh, “Large Libel Models? Liability for AI Output” at page 500.
#3: Allocating liability to incentivise the development and deployment of LLMs with more accurate output?
Turning to the developer/deployer, liability should be assessed across three scenarios based on the cases reported in the news so far – the outcomes do differ based on the scenario:
- Scenario 1: in the case of output that is wrong but harmless/trivial (e.g. relying on the answer would not have affected a person’s rights, such as the right to make a claim for compensation),30See the example of an airline’s customer who praised a flight attendant for helping her to “take care of a plant cutting” and was referred to a suicide prevention hotline by the airline’s AI-enabled chatbot as it had flagged the word “cutting” – https://www.cbc.ca/news/canada/calgary/westjet-ai-chatbot-confusion-suicide-hotline-1.4836389 or that is so clearly wrong that no one can reasonably be expected to have relied on it – the harm is de minimis and merely seen as an amusing side effect of the technology, with no one being held liable;
- Scenario 2: in the case of wrong output that a person relied on to their detriment – the question is whether the person who offered31E.g. a company has a chatbot on its website, thus “offering” and making the technology available to users – see case of Air Canada discussed below. or used32E.g. a person (Person A) uses generative AI to create a work product, and then submits that work product on to a third party (Person B) that Person A has a duty to submit (correct) information to. This would be cases of lawyers using ChatGPT to prepare submissions to court without checking the content generated, discussed below. the technology to generate output was under any duty to ensure that the recipient of the output received the correct information;
- Scenario 3: in the case of defamatory content – would this in part depend on the prompt, who viewed the output, as well as the reaction of the developer/deployer when informed of the defamatory output (e.g. they put in place filters to ensure such content is not repeated)?
One might be tempted to take an approach for deployers/developers that “if you use it, you are liable for it if the risk is foreseeable”. The risks (wrong content/toxic content) are clearly foreseeable since developers/deployers mention them in their disclaimers. However, it is important to determine liability a fair and balanced way so that there is no chilling effect on the industry.33This is especially since there is a huge push in Singapore for SMEs to utilise generative AI solutions like generative AI-powered chatbots to allow customers to search for information, browse recommendations personalised for them, and make reservations – see https://www.imda.gov.sg/resources/press-releases-factsheets-and-speeches/press-releases/2024/sg-first-genai-sandbox-for-smes.
Scenario 1: Trivial wrongs, regardless of whether there is a duty to give correct information to the person receiving output from the LLM
If the output is so clearly wrong that no sensible person would have relied on it (e.g. “the moon is made of cheese”), the public consensus appears to be that no one should be liable. A search on the Internet has not revealed any lawsuits for such scenarios to date.34Searches surface the Mark Walters and Australian Mayor cases only, which relate to defamation.
This is similar to the approach taken where text-to-image generators generate the wrong images which are not toxic in and of themselves (e.g. not depicting pornography or violent content). Google’s Gemini image generator was criticised for producing historically inaccurate images, such as medieval British kings of different genders and races.35As reported in https://www.cnbc.com/2024/02/26/googles-gemini-ai-picture-generator-to-relaunch-in-a-few-weeks.html. But end of the day, such text-to-image generators are not a search engine for (existing) images where the expectation is that they will be historically accurate – they are creativity tools generating new images. Therefore, no liability has arisen for developers to date, aside from press coverage that may not necessarily be flattering, and warnings to people to be careful when using the image generators. Google has responded by turning off Gemini’s function to generate images of people to improve it before re-releasing it.36https://blog.google/products/gemini/gemini-image-generation-issue/
It is suggested that reputational risk will keep developers in check, and they will try to minimise instances where their LLMs/text-to-image generators give wrong output to gain an edge over the product of their competitors. It is unlikely that the developer will be liable for such trivial wrongs unless the developer had made certain promises to its customers (i.e. deployers) to implement technological solutions and it is found that the developer did not – in which case it is for the lack of processes/diligence rather than the output.
Scenario 2: An LLM is used to give information by a person who has a duty to the recipient of the information to ensure the information given to them is correct, as the recipient will suffer detriment with the wrong information
There have been cases where deployers (not developers) are liable for wrong information communicated by chatbots – this is where the deployer has a duty to the recipient of the information to ensure that the information provided to the recipient is correct.
Relationship 1: Airline and customer seeking information on airline’s policies through chatbot on airline’s website37Moffatt v Air Canada, 2024 BCCRT 149, accessible at https://www.canlii.org/en/bc/bccrt/doc/2024/2024bccrt149/2024bccrt149.html
A customer had a query on bereavement fares and used a chatbot on Air Canada’s website. The chatbot gave the customer the wrong information in its response:
- the chatbot stated that reduced bereavement rates could apply retroactively (i.e. the customer could travel first on a full-priced ticket and be refunded the difference after), but in actual fact the customer had to claim the bereavement rate before travelling.
[note: in contrast to Scenario 1, the chatbot’s response was a plausible answer, and not one that would appear at first blush to the customer to be so clearly wrong];
- the chatbot also gave a link to the airline’s bereavement fare policy page which contained information contradictory to the chatbot’s response – that bereavement fares would not cover travel that has already been completed.
Air Canada responded that it should not be liable for the information communicated by the chatbot; that the chatbot was a “separate legal entity that is responsible for its own actions”.38Moffatt v Air Canada at (27). The tribunal disagreed, saying that “[w]hile a chatbot has an interactive component, it is still just a part of Air Canada’s website. It should be obvious to Air Canada that it is responsible for all the information on its website. It makes no difference whether the information comes from a static page or a chatbot.”.39Moffatt v Air Canada at (27).
The judgment did not cover whether there was a disclaimer that the chatbot may give inaccurate information on occasion. Nevertheless, if there was, would the disclaimer have been effective?
The tribunal found that Air Canada “did not take reasonable care to ensure its chatbot was accurate”,40Moffatt v Air Canada at (28). and the tribunal accepted that it was “reasonable in the circumstances” that the customer “relied upon the chatbot to provide accurate information”.41Moffatt v Air Canada at (29). Even if a link to another part of the website containing the (correct) information was provided by the chatbot to the customer, customers were not expected to have to double-check information received, or know that one section of the website was accurate while another was not.42Moffatt v Air Canada at (28).
The answer is thus inconclusive – but practically, putting such a disclaimer up would defeat the convenience purpose of having such a chatbot in the first place, if the customer still has to scour the website to find/cross-check the information required.
Relationship 2: Person (who uses LLM to generate output) with duty to ensure that the information that person provides to a third party is correct
Another instance is if the person who uses the LLM has a duty to provide correct information to a third party – such as when a lawyer is presenting information to the court. The lawyer ultimately remains responsible for any work product issued in his or her name, whether written by himself/herself, his/her associates or by a generative AI application, and errant lawyers have been sanctioned.43In addition to Roberto Mata v Avianca, Inc., see also the latest case of Darlene Smith v Matthrew Farwell & Ors, accessible at https://masslawyersweekly.com/wp-content/blogs.dir/1/files/2024/02/12-007-24.pdf The lawyers’ lack of awareness/knowledge that LLMs hallucinate was not a defence.
Scenario 3: Defamatory content
In relation to the developer/deployer, is there a duty to ensure that the LLM application does not generate content that causes people reputational harm?
There is no case yet in Singapore. Internationally, the Mark Walters lawsuit (of June 2023) in the USA is still ongoing, and as of January 2024 the court denied OpenAI’s motion to dismiss it. In contrast, the Australian mayor dropped his bid to sue OpenAI in February 2024 since OpenAI had corrected the material output in the latest version of ChatGPT (and he also acknowledged some difficulties in proving his defamation claim).44As reported in https://www.smh.com.au/technology/australian-mayor-abandons-world-first-chatgpt-lawsuit-20240209-p5f3nf.html; the newspaper described that this would otherwise have been a “world first test case over false claims made by an AI chatbot”. Nevertheless, one would say that the mayor taking his case to the press had cleared his name more effectively than any lawsuit could have!
Legal experts cannot agree45Contrasting views are presented in this article, available at https://arstechnica.com/tech-policy/2023/06/openai-sued-for-defamation-after-chatgpt-fabricated-yet-another-lawsuit/ on whether developers/deployers should be liable for the LLM’s defamatory output. The answer is unlikely to be a straightforward yes/no as it would depend on many factors (and defamation laws vary by jurisdiction):
- the output produced in part depends on the prompt by the user – e.g. if asked to write about a sexual offence that Person A committed, even if there was none, the model might well come up with something;46Peter Henderson, Tatsunori Hasimoto & Mark Lemley, “Where’s the Liability in Harmful AI Speech?”, published in the Journal of Free Speech Law, August 2023, at page 596.
- it may not be realistic to expect developers to calibrate their models to “only say the good stuff about people”, as that would make the information incorrect/omit pertinent negative information that is true about a person;47Peter Henderson, Tatsunori Hasimoto & Mark Lemley, “Where’s the Liability in Harmful AI Speech?” at page 647.
- the LLM could have been trained on false information about a person, leading it to produce the false output when prompted – but it is neither practical nor realistic to expect developers or deployers to verify all training data;
- the extent of publication of the output48As acknowledged by the Australian mayor in withdrawing his lawsuit (see https://www.smh.com.au/technology/australian-mayor-abandons-world-first-chatgpt-lawsuit-20240209-p5f3nf.html). See also Professor Eugene Volokh’s commentary that Mark Walters’ complaint “doesn’t appear to meet the relevant standards under defamation law. Walters never claimed he told OpenAI that ChatGPT was making fake allegations. The fact that (the person who entered the prompt that produced the results) never published the falsehood would likely limit the economic damages Walters could prove.”, as reported in https://news.bloomberglaw.com/ip-law/first-chatgpt-defamation-lawsuit-to-test-ais-legal-liability. – technically it is only seen by the person who entered the prompt (but if the prompter posts the output online, the prompter is taken to have endorsed/adopted that statement as his or her own and can be liable for it). Therefore, if I prompt ChatGPT and get defamatory output about myself, the harm is de minimis as only I have read it. However, I would be rightly concerned if others ask ChatGPT about me and they also got a false/defamatory response – this was the case for Mark Walters, the Australian Mayor and Jonathan Turley – the output from ChatGPT was brought to their attention by third parties/friends. How the court rules in the Mark Walters case will be instructive.
Part 2: Liability for Toxic Output
The other type of content we will focus on is content that is toxic – think content that would be an offence to share publicly under our existing laws, such as content promoting violence, sexually explicit content, etc.49This does not include content that is ‘legal but harmful’ – e.g. content promoting dieting for youths. A notable example was Microsoft’s Tay chatbot which users tricked into posting racist and offensive content.50As reported in https://www.cbsnews.com/news/microsoft-shuts-down-ai-chatbot-after-it-turned-into-racist-nazi/.
A subset of this content liability would be deepfakes. Deepfakes are images/videos that have been manipulated to replace a real person’s likeness convincingly with another person’s – and can be used in a multitude of situations, from scams to the distribution of explicit images.51See the definition of “deepfake” in the Oxford English Dictionary at https://www.oed.com/dictionary/deepfake_n?tl=true. See also an article explaining what are deepfakes at https://www.theguardian.com/technology/2020/jan/13/what-are-deepfakes-and-how-can-you-spot-them. They are also distinguished from “wrong” output outlined in Part 1 because such content is an unintended side-effect of LLMs, as opposed to being deliberately generated by a person.
What are the Current Solutions?
#1: Content filters and other technological solutions
Some of the current technical solutions recommended in Singapore’s Model Gen-AI Framework for both text generators and text-to-image generators are:
- both the prompt and the output will be run through filters52See page 10 of the Model Gen-AI Framework: “For example, further fine-tuning or using user interaction techniques (such as input and output filters) can help to reduce harmful output. Techniques like Retrieval-Augmented Generation (RAG) and few-shot learning are also commonly used to reduce hallucinations and improve accuracy.” (in essence a series of programmed rules) that will detect potentially harmful content across defined categories like hate, sexual content, violence and self-harm – if the prompt is deemed inappropriate there would not be a response, or the response will be filtered.53In relation to LLMs, for example, Microsoft’s Azure OpenAI Service has a content filtering system that runs the prompt and the completion through content filtering models that have been trained to detect language promoting hate, sexual content, violence and self-harm in a variety of languages. There are 4 levels of severity of the content – safe, low, medium and high, where the default content filtering configuration is for medium-severity content. Read more at https://learn.microsoft.com/en-us/azure/ai-services/openai/concepts/content-filter?tabs=warning%2Cpython. In relation to text-to-image generators, read more about the filters at https://techxplore.com/news/2023-11-filter-tackle-unsafe-ai-generated-images.html However, the right balance must be struck – Singapore’s IMDA has cautioned that “it is not as simple as just filtering or checking against toxic content. A naïve filter for generative AI that refuses to answer a prompt like ‘The Holocaust was …’ risks censoring useful information.”54See page 11 of IMDA’s Generative AI Risks Paper.
- Red-teaming, where the evaluators act as adversarial users to “break” the model and attempt to induce safety, security and other violations, so that corrective action can be taken.55See page 11 of the Model Gen-AI Framework. But red-teaming also has its limitations, as the quality of a red-teaming evaluation is dependent on the expertise and impartiality of the team that conducts it – “a team lacking in skill or hampered by biases may fail to rigorously probe (a generative AI model’s) vulnerabilities, thereby inducing a false sense of security” (see paragraph 29 of IMDA’s Cataloguing LLM Evaluations paper).
However, it is widely acknowledged that such methods cannot eliminate toxic or unwanted output entirely.56For example, the UK acknowledges that “(s)ince it is not possible to build models that never produce unwanted or fictitious outputs (i.e. hallucinations), incorporating end-user feedback is vital. Put mechanisms into place that allow end-users to report content and trigger a human review process.” – see Principle 4 on page 10 of the Generative AI framework for HM Government created by the UK’s Central Digital and Data Office.
#2: Laws/recommendations on what training data can be used (the idea being that if a model is not trained on harmful data, its outputs are less likely to be harmful)
On 11 October 2023, China launched a public consultation (until 25 October) on blacklisting of training datasets of AI models, where data sources with more than 5% of illegal and harmful information (e.g. containing information censored on the Chinese web, or promoting terrorism or violence) are blacklisted and cannot be used to train AI models.57As reported in https://www.channelnewsasia.com/business/china-proposes-blacklist-training-data-generative-ai-models-3841431 How this is calculated is to randomly sample 4,000 “pieces” of data from one source, and if more than 5% of the data is considered illegal/harmful, that source should not be used for training.58As explained in https://www.technologyreview.com/2023/10/18/1081846/generative-ai-safety-censorship-china To date, no law has been enacted yet.
China is not alone in this – Singapore59See page 9 of the Model Gen-AI Framework. is also advocating the development of “[a] pool of trusted data sets” that “reflect the cultural and social diversity of a country” so that “safer and more culturally representative models” will be developed. Developers are also taking pains to emphasise what data is used in training their models – e.g. OpenAI states that they “apply filters and remove information that [they] do not want [their] models to learn from or output, such as hate speech, adult content, sites that primarily aggregate personal information, and spam”.60See https://help.openai.com/en/articles/7842364-how-chatgpt-and-our-language-models-are-developed (last accessed 10 March 2024).
Nevertheless, this solution is also limited as there is research that shows that harmful outputs “may arise even when the model never trains on any one problematic text. In effect, it can hallucinate new harmful behaviour, not grounded in anything it has seen before”.61Peter Henderson, Tatsunori Hasimoto & Mark Lemley, “Where’s the Liability in Harmful AI Speech?”, at page 593.
#3: Warnings to users that output generated may be toxic/inappropriate, and a mechanism to report such output, tied together with acceptable use policies
As discussed earlier in relation to inaccurate content, developers/deployers of generative AI services have disclaimers, warning that the service may provide “inaccurate or offensive” output, with mechanisms for users to report such output.62For example, Google has a form for users to report “any generated output that contains inappropriate material or inaccurate information” (https://cloud.google.com/vertex-ai/generative-ai/docs/learn/responsible-ai under the “Report abuse” section). These are also bundled with acceptable use policies instructing users to comply with applicable laws, not to use the services to harm others (e.g. defraud, scam, promote violence or hatred), and not to circumvent the technical safeguards built into the services.63See, for example, Open AI’s universal usage policies at https://openai.com/policies/usage-policies.
This trio alone is not sufficient to extinguish any liability that may arise on the part of the developer or deployer, but it is necessary to complement the technological solutions mentioned in #1 above so that users are aware of how they are to use the generative AI service.
#4: Should developers/deployers be liable for toxic content generated by generative AI, to ensure more responsible model development?
One of the most prominent debates on whether there should be immunity for AI-generated content can be found in the USA. There is ambiguity64As reported in https://cbsaustin.com/news/nation-world/regulating-ai-could-require-fundamental-change-in-law-artificial-intelligence-section-230-social-media-big-tech-congress-republican-democrat-bipartisan-legislation-defamation-lawsuit. See also the commentary from one of the original drafters of section 230 (back in 1996) who opined that it would not cover generative AI – https://www.marketplace.org/shows/marketplace-tech/section-230-co-author-says-the-law-doesnt-protect-ai-chatbots/. over whether section 230 of the Communications Decency Act65We did not discuss section 230 in relation to trivial wrong content because section 230 was enacted to cover defamatory, harmful or illegal content. There should be something inherently morally wrong about the output rather than it just being inaccurate. See an explainer of section 230 at https://itif.org/publications/2021/02/22/overview-section-230-what-it-why-it-was-created-and-what-it-has-achieved/. applies to generative AI output – the case of Google v Gonzalez had Justice Gorsuch opine that section 230 would not apply to generative AI as it created new content – it “goes beyond picking, choosing, analysing, or digesting content”66Justice Gorsuch’s remarks were reported in https://cbsaustin.com/news/nation-world/regulating-ai-could-require-fundamental-change-in-law-artificial-intelligence-section-230-social-media-big-tech-congress-republican-democrat-bipartisan-legislation-defamation-lawsuit. Since then, there have been movements in the USA to expressly remove that immunity: a “No Section 230 Immunity for AI Act” was proposed, but the Senate has since rejected it.
In Singapore (and ASEAN), this issue is still under consideration but is a priority – ASEAN intends “for policymakers to facilitate and co-create with developers a shared responsibility framework” that will “clarify the responsibilities of all parties in the AI system life cycle, as well as the safeguards and measures they need to respectively undertake.”67See page 56 of the ASEAN Guide on AI Governance and Ethics.
In the meantime (until we have a test case), if a developer/deployer has done all it reasonably can to minimise harmful output from the generative AI system given the level of technology available — (1) implemented technological safeguards commonly used in the industry such as content filters; (2) filtered the training data used; (3) set out content warnings and acceptable use policies for users, with mechanisms to report any toxic content generated – a good case can be made that it should not be liable for the harmful output. This is especially if the user deliberately prompted the AI system to generate such toxic content. The converse would apply if the developer/deployer had deliberately/purposefully programmed the AI system to produce harmful content.
The views expressed in this article are the personal views of the author and do not represent the views of Drew & Napier LLC.
Endnotes
↑1 | The six risks posed by generative AI were identified and discussed in the Infocomm Media Development Authority’s paper on “Generative AI: Implications for Trust and Governance” published on 6 June 2023 (“Generative AI Risks Paper”), affirmed again by ASEAN in February 2024 in the ASEAN Guide on AI Governance and Ethics – of which this article will focus on these 2 risks. |
---|---|
↑2 | This is the first risk identified in IMDA’s Generative AI Risks Paper, which acknowledges that all generative AI models will make mistakes (page 9). |
↑3 | As described in Google’s model limitations for its generative AI products, available at https://cloud.google.com/vertex-ai/generative-ai/docs/learn/responsible-ai |
↑4 | This is the third risk identified in IMDA’s Generative AI Risks Paper, which remains a challenge so long as generative models “mirror language from the web” (page 11). |
↑5 | For information about how LLMs work, read more at https://developers.google.com/machine-learning/resources/intro-llms. |
↑6 | See the US Copyright Review Board’s decision on Théâtre D’opéra Spatial dated 5 September 2023, in particular pages 6 and 7, available at https://www.copyright.gov/rulings-filings/review-board/docs/Theatre-Dopera-Spatial.pdf. |
↑7 | The definition of “developer” is taken from the ASEAN Guide on AI Governance and Ethics (page 9). |
↑8 | See the distinction between AI developers and deployers at https://www.bsa.org/files/policy-filings/03162023aidevdep.pdf. |
↑9 | The definition of “deployer” is adapted from the ASEAN Guide on AI Governance and Ethics (page 9). |
↑10 | Please note that an in-depth look into how to allocate liability as between developer and deployer is outside the scope of this article (in order to remain within the word limit). However, the following is a useful resource on the allocation of liability depending on how the model is made accessible to a developer downstream (via API or open source): https://www.adalovelaceinstitute.org/blog/value-chain-general-purpose-ai/. Singapore’s proposed Model AI Governance Framework for Generative AI (issued on 16 January 2024) also puts forth the proposition that “responsibility can be allocated based on the level of control that each stakeholder has in the generative AI development chain, so that the able party takes necessary action to protect end-users.” (p. 6). |
↑11 | The definition of “user” is adapted from the ASEAN Guide on AI Governance and Ethics (page 9), but in this case there is no “decision” made by the generative AI system. |
↑12 | This excludes scenarios where the user is legitimately engaged by the developer or deployer to test the AI system (e.g. red-teaming). |
↑13 | Potential laws that could apply would include the Penal Code 1871 (e.g. for distributing intimate images, or for cheating (e.g. a deepfake is used in scams)); the Protection from Harassment Act 2014, the Undesirable Publications Act 1967 and the Protection from Online Falsehoods and Manipulation Act 2019 (“POFMA”). |
↑14 | Separately, see also section 11(4) of the POFMA where a person who communicates a false statement of fact in Singapore may be issued a Correction Direction even if the person does not know or has no reason to believe that the statement is false. The High Court in The Online Citizen Pte Ltd v Attorney-General (2020) SGHC 36 explained that “Section 11(4) of the POFMA is intended to prevent and stop the spread of falsehoods and misleading information when information is posted online without prior verification and thought, be it deliberate or otherwise.” |
↑15 | See Roberto Mata v Avianca, Inc., as reported in https://www.theverge.com/2023/5/27/23739913/chatgpt-ai-lawsuit-avianca-airlines-chatbot-research |
↑16 | As reported in https://www.smh.com.au/technology/australian-whistleblower-to-test-whether-chatgpt-can-be-sued-for-lying-20230405-p5cy9b.html |
↑17 | As reported in https://nypost.com/2023/04/07/chatgpt-falsely-accuses-law-professor-of-sexual-assault/ |
↑18 | https://nypost.com/2023/06/07/mark-walters-suing-chatgpt-for-embezzled-hallucination/, where the New York Post also described it as the “first-ever defamation lawsuit” against OpenAI. |
↑19 | As quoted in https://www.straitstimes.com/world/europe/british-judge-taps-jolly-useful-chatgpt-for-part-of-his-ruling#:~:text=A%20British%20Court%20of%20Appeal,Lord%20Justice%20Colin%20Birss%20said. |
↑20 | As quoted in https://www.straitstimes.com/world/europe/british-judge-taps-jolly-useful-chatgpt-for-part-of-his-ruling#:~:text=A%20British%20Court%20of%20Appeal,Lord%20Justice%20Colin%20Birss%20said. |
↑21 | There are many more technological solutions, but I will focus on those mentioned in discussion papers issued by IMDA to date, namely the Generative AI Risks Paper (issued in June 2023), the Cataloguing LLM Evaluations paper (issued in October 2023), and the proposed Model AI Governance Framework for Generative AI (issued on 16 January 2024). |
↑22 | See page 19 of the Model Gen-AI Framework: “For example, further fine-tuning or using user interaction techniques (such as input and output filters) can help to reduce harmful output. Techniques like Retrieval-Augmented Generation (RAG) and few-shot learning are also commonly used to reduce hallucinations and improve accuracy.” |
↑23 | Adapting the example provided by Moveworks in https://www.youtube.com/watch?v=6dxkBftbukI. |
↑24 | See page 13 of IMDA’s Generative AI Risks Paper, which states that with RHLF, “language models learn to follow instructions better and generate results that show fewer instances of “hallucination” and toxicity (even though bias still remains as an open problem)”. See also the concept of “chain-of-thought prompting” mentioned in page 22 of IMDA’s Generative AI Risks Paper, as a means to improve model quality with better explainability through reasoning. It involves providing the LLM with a question and also the method to solve it, so that the LLM will not only give the answer but also show its reasoning for the answer, increasing the likelihood of getting the correct answer – read more about chain-of-thought prompting at https://blog.research.google/2022/05/language-models-perform-reasoning-via.html. |
↑25 | See, for example, the Generative AI framework for HM Government created by the UK’s Central Digital and Data Office at page 10, accessible at: https://assets.publishing.service.gov.uk/media/65c3b5d628a4a00012d2ba5c/6.8558_CO_Generative_AI_Framework_Report_v7_WEB.pdf. See also the interviews at https://fortune.com/2023/08/01/can-ai-chatgpt-hallucinations-be-fixed-experts-doubt-altman-openai/. |
↑26 | See, for example, Google’s Disclaimer at https://cloud.google.com/terms/service-terms, which states that “Generative AI Services use emerging technology, may provide inaccurate or offensive Generated Output, and are not designed for or intended to meet Customer’s regulatory, legal or other obligations.” |
↑27 | As reported in https://futurism.com/the-byte/judge-refuses-dismiss-libel-suit-openai and https://www.insideradio.com/free/another-setback-for-openai-in-radio-hosts-defamation-suit/article_ffb78558-b583-11ee-a92d-53d6ae459450.html |
↑28 | Eugene Volokh, Professor of Law at UCLA School of Law, in “Large Libel Models? Liability for AI Output” published in the Journal of Free Speech Law, August 2023 (at pages 501 and 498 to 499). |
↑29 | Eugene Volokh, “Large Libel Models? Liability for AI Output” at page 500. |
↑30 | See the example of an airline’s customer who praised a flight attendant for helping her to “take care of a plant cutting” and was referred to a suicide prevention hotline by the airline’s AI-enabled chatbot as it had flagged the word “cutting” – https://www.cbc.ca/news/canada/calgary/westjet-ai-chatbot-confusion-suicide-hotline-1.4836389 |
↑31 | E.g. a company has a chatbot on its website, thus “offering” and making the technology available to users – see case of Air Canada discussed below. |
↑32 | E.g. a person (Person A) uses generative AI to create a work product, and then submits that work product on to a third party (Person B) that Person A has a duty to submit (correct) information to. This would be cases of lawyers using ChatGPT to prepare submissions to court without checking the content generated, discussed below. |
↑33 | This is especially since there is a huge push in Singapore for SMEs to utilise generative AI solutions like generative AI-powered chatbots to allow customers to search for information, browse recommendations personalised for them, and make reservations – see https://www.imda.gov.sg/resources/press-releases-factsheets-and-speeches/press-releases/2024/sg-first-genai-sandbox-for-smes. |
↑34 | Searches surface the Mark Walters and Australian Mayor cases only, which relate to defamation. |
↑35 | As reported in https://www.cnbc.com/2024/02/26/googles-gemini-ai-picture-generator-to-relaunch-in-a-few-weeks.html. |
↑36 | https://blog.google/products/gemini/gemini-image-generation-issue/ |
↑37 | Moffatt v Air Canada, 2024 BCCRT 149, accessible at https://www.canlii.org/en/bc/bccrt/doc/2024/2024bccrt149/2024bccrt149.html |
↑38 | Moffatt v Air Canada at (27). |
↑39 | Moffatt v Air Canada at (27). |
↑40 | Moffatt v Air Canada at (28). |
↑41 | Moffatt v Air Canada at (29). |
↑42 | Moffatt v Air Canada at (28). |
↑43 | In addition to Roberto Mata v Avianca, Inc., see also the latest case of Darlene Smith v Matthrew Farwell & Ors, accessible at https://masslawyersweekly.com/wp-content/blogs.dir/1/files/2024/02/12-007-24.pdf |
↑44 | As reported in https://www.smh.com.au/technology/australian-mayor-abandons-world-first-chatgpt-lawsuit-20240209-p5f3nf.html; the newspaper described that this would otherwise have been a “world first test case over false claims made by an AI chatbot”. Nevertheless, one would say that the mayor taking his case to the press had cleared his name more effectively than any lawsuit could have! |
↑45 | Contrasting views are presented in this article, available at https://arstechnica.com/tech-policy/2023/06/openai-sued-for-defamation-after-chatgpt-fabricated-yet-another-lawsuit/ |
↑46 | Peter Henderson, Tatsunori Hasimoto & Mark Lemley, “Where’s the Liability in Harmful AI Speech?”, published in the Journal of Free Speech Law, August 2023, at page 596. |
↑47 | Peter Henderson, Tatsunori Hasimoto & Mark Lemley, “Where’s the Liability in Harmful AI Speech?” at page 647. |
↑48 | As acknowledged by the Australian mayor in withdrawing his lawsuit (see https://www.smh.com.au/technology/australian-mayor-abandons-world-first-chatgpt-lawsuit-20240209-p5f3nf.html). See also Professor Eugene Volokh’s commentary that Mark Walters’ complaint “doesn’t appear to meet the relevant standards under defamation law. Walters never claimed he told OpenAI that ChatGPT was making fake allegations. The fact that (the person who entered the prompt that produced the results) never published the falsehood would likely limit the economic damages Walters could prove.”, as reported in https://news.bloomberglaw.com/ip-law/first-chatgpt-defamation-lawsuit-to-test-ais-legal-liability. |
↑49 | This does not include content that is ‘legal but harmful’ – e.g. content promoting dieting for youths. |
↑50 | As reported in https://www.cbsnews.com/news/microsoft-shuts-down-ai-chatbot-after-it-turned-into-racist-nazi/. |
↑51 | See the definition of “deepfake” in the Oxford English Dictionary at https://www.oed.com/dictionary/deepfake_n?tl=true. See also an article explaining what are deepfakes at https://www.theguardian.com/technology/2020/jan/13/what-are-deepfakes-and-how-can-you-spot-them. |
↑52 | See page 10 of the Model Gen-AI Framework: “For example, further fine-tuning or using user interaction techniques (such as input and output filters) can help to reduce harmful output. Techniques like Retrieval-Augmented Generation (RAG) and few-shot learning are also commonly used to reduce hallucinations and improve accuracy.” |
↑53 | In relation to LLMs, for example, Microsoft’s Azure OpenAI Service has a content filtering system that runs the prompt and the completion through content filtering models that have been trained to detect language promoting hate, sexual content, violence and self-harm in a variety of languages. There are 4 levels of severity of the content – safe, low, medium and high, where the default content filtering configuration is for medium-severity content. Read more at https://learn.microsoft.com/en-us/azure/ai-services/openai/concepts/content-filter?tabs=warning%2Cpython. In relation to text-to-image generators, read more about the filters at https://techxplore.com/news/2023-11-filter-tackle-unsafe-ai-generated-images.html |
↑54 | See page 11 of IMDA’s Generative AI Risks Paper. |
↑55 | See page 11 of the Model Gen-AI Framework. But red-teaming also has its limitations, as the quality of a red-teaming evaluation is dependent on the expertise and impartiality of the team that conducts it – “a team lacking in skill or hampered by biases may fail to rigorously probe (a generative AI model’s) vulnerabilities, thereby inducing a false sense of security” (see paragraph 29 of IMDA’s Cataloguing LLM Evaluations paper). |
↑56 | For example, the UK acknowledges that “(s)ince it is not possible to build models that never produce unwanted or fictitious outputs (i.e. hallucinations), incorporating end-user feedback is vital. Put mechanisms into place that allow end-users to report content and trigger a human review process.” – see Principle 4 on page 10 of the Generative AI framework for HM Government created by the UK’s Central Digital and Data Office. |
↑57 | As reported in https://www.channelnewsasia.com/business/china-proposes-blacklist-training-data-generative-ai-models-3841431 |
↑58 | As explained in https://www.technologyreview.com/2023/10/18/1081846/generative-ai-safety-censorship-china |
↑59 | See page 9 of the Model Gen-AI Framework. |
↑60 | See https://help.openai.com/en/articles/7842364-how-chatgpt-and-our-language-models-are-developed (last accessed 10 March 2024). |
↑61 | Peter Henderson, Tatsunori Hasimoto & Mark Lemley, “Where’s the Liability in Harmful AI Speech?”, at page 593. |
↑62 | For example, Google has a form for users to report “any generated output that contains inappropriate material or inaccurate information” (https://cloud.google.com/vertex-ai/generative-ai/docs/learn/responsible-ai under the “Report abuse” section). |
↑63 | See, for example, Open AI’s universal usage policies at https://openai.com/policies/usage-policies. |
↑64 | As reported in https://cbsaustin.com/news/nation-world/regulating-ai-could-require-fundamental-change-in-law-artificial-intelligence-section-230-social-media-big-tech-congress-republican-democrat-bipartisan-legislation-defamation-lawsuit. See also the commentary from one of the original drafters of section 230 (back in 1996) who opined that it would not cover generative AI – https://www.marketplace.org/shows/marketplace-tech/section-230-co-author-says-the-law-doesnt-protect-ai-chatbots/. |
↑65 | We did not discuss section 230 in relation to trivial wrong content because section 230 was enacted to cover defamatory, harmful or illegal content. There should be something inherently morally wrong about the output rather than it just being inaccurate. See an explainer of section 230 at https://itif.org/publications/2021/02/22/overview-section-230-what-it-why-it-was-created-and-what-it-has-achieved/. |
↑66 | Justice Gorsuch’s remarks were reported in https://cbsaustin.com/news/nation-world/regulating-ai-could-require-fundamental-change-in-law-artificial-intelligence-section-230-social-media-big-tech-congress-republican-democrat-bipartisan-legislation-defamation-lawsuit |
↑67 | See page 56 of the ASEAN Guide on AI Governance and Ethics. |